Generating Insights from Data - Citizen Data Scientist
- 0.5
- 1
- 1.25
- 1.5
- 1.75
- 2
Nick Einstein: Welcome, thanks for joining us today. It's a pleasure to dig into democratizing data, machine learning and Cheetah's Citizen Data Scientist. My name is Nick Einstein, I'm in product marketing. I'm joined today by Schuyler Wareham, who's senior director of product management. Today, we're going to be talking about machine learning and Cheetah's Citizen Data Scientist. Schuyler, thanks for being here.
Schuyler Wareham: Great to be here, Nick. Thank you.
Nick Einstein: This is a hot topic. We've talked about it before and I always like kicking it off by grounding us a little bit in why the topic is so critical today. The bottom line is your customers are spending a greater percentage of their time online than ever before in their lives, across all their devices, their phones, their laptops, their tablets, even their televisions, and spending more through e- commerce as well. They're generating two megabytes of customer data every second, over 2. 5 quintillion bytes of data every day and that number is growing exponentially. As marketers, you need to effectively leverage those data because your customers have high expectations. They receive over a hundred emails a day and on average, nearly 50 text messages and they ignore those that don't provide recognizable value to them. In order to delight your customers, you as marketers need to deliver personalized experiences that harness all the rich data that's been generated across their customer journey and do so at the precise moments that matter most. In many instances, this requires machine learning and data science to make it happen. As the Harvard Business Review recently said," If data science is to be truly transformational, everyone must get in on the fun. Restricting data science to only the experts is a limiting proposition, data science programs that focus on professional data scientists ignore the vast of people and business opportunities." Schuyler, let's talk a little bit more about that now, maybe unpack that a bit. Schuy, what is citizen data science and what is a citizen data scientist?
Schuyler Wareham: Well, let's level set with Gartner's definition. Don't take it from me personally," Citizen data science is an emerging set of capabilities and practices that allow users to extract predictive and prescriptive insights from data while not requiring them to be as skilled or technically sophisticated as expert data scientists. So therefore, it follows that a citizen data scientist is a person who creates or generates models that use advanced diagnostic analytics, predictive and prescriptive capabilities, but whose primary job function is outside the field of statistics and analytics."
Nick Einstein: Okay, those are the Gartner definitions, Schuy. What is the Cheetah Digital Citizen Data Scientist product?
Schuyler Wareham: Our manifestation of Citizen Data Scientist is to provide marketers with a simple Gooey- based workflow, that a marketer can go through to build a custom model per their predictive use cases, with clicks and no code and no advance knowledge of what statistical techniques need to be deployed to solve for those use cases. They can integrate any customer data and deploy models against any target, outcome variable of interest, assuming that that data resides within our platform. It can incorporate model outcomes in predictions to a more robust customer profile that drives engagements and personalization strategies.
Nick Einstein: And what can citizen data scientists do? What questions can they answer?
Schuyler Wareham: Well, at a really high level, what ML and AI can do for the marketer is to improve common tasks. The efficiency and operationalization of common tasks surface hidden insights. It's one thing to simply provide a score for every customer record, but it's quite another for the marketer to be able to digest and internalize the drivers of the predictive factors, or the profile characteristics that comprise someone who is likely or unlikely to take some sort of action. It can generate next best actions, so recommendations for offers or products. Then finally it's always on, it's always learning. It really takes the burden off of the human and these machine learning models can be retrained and rescored on a regular cadence without any sort of manual or hands- on intervention.
Nick Einstein: A lot of use cases, a lot of critical use cases across the enterprise.
Schuyler Wareham: So, here's a good takeaway slide, but I'll just touch on a handful of the bullet points. So, we have really three main categories that we cover, predictive models, segmentation, and send time optimization. There are some others here listed. Under the predictive model pillar, we can do things like activity scoring. I alluded to predicting the likelihood that someone is likely to do X, Y, or Z. We could also provide lookalike targeting, so helpful for top of the funnel, prospecting, lead generation and what have you, and also on the flip side, predicting churn or attrition. So, every business has attrition and predictive models can help be an early warning system for identifying who might be at risk of churning and what the factors are that contribute to that likelihood. On the segmentation side, I think most people are familiar with clustering. So, identifying groups of members or individuals that have similar characteristics, behaviors, and attributes, but who are distinct from other clusters with their own set of profile characteristics. Offer optimization or recommendation system that prioritizes and personalizes offers is another full technically, of segmentation. And then we have send time optimization. So, identifying in a prescriptive way when to communicate to a given individual, where they'll have the greatest likelihood of engaging with that content.
Nick Einstein: Take us into what we do have in the platform in the way of machine learning.
Schuyler Wareham: Yeah, so we have with respect to out- of- the- box models and Citizen Data Scientist capabilities, propensity modeling, where the machine learning is listening and analyzing customer behavior and characteristics, and identifying the likelihood that a given out outcome will occur. In the out- of- the- box examples, it's to open, to click, to un- engage with your marketing program. With Citizen Data Scientist examples, it can be other custom outcomes or activities that you would specify. Secondly, we have cluster models. So as I alluded to, these are algorithms that automatically identify natural groupings of customers who behave similarly and share common characteristics and produce audience segments that can be used for targeting, and then finally send time optimization. Okay, so here we are at the home for machine learning within the Cheetah EDP, and I'm going to show you how quick and easy it to create a custom Citizen Data Scientist model. So, let's start with a propensity example, actually two propensity examples, a two for one, Signals21 CDS Demo. So, I've simply given it a name here and I'm going to click Create Citizen Model and up pops this modal. Here at the top, you'll see the five step process to create a Citizen Data Scientist model. We've already given it a name and there a couple of other items here that you can use just to set the stage. The first is specifying a segment. So within the EDP, you can create many, many segments with your specified criteria. Those segments can then be used to focus the model in on a particular audience. Similarly, with business units, if you have a parent and child setup, you can focus in on particular brand or another, or the entire enterprise. Now define outcome. Second step here is one of the more critical ones. So, here is where we can predict a future marketing event, a future activity. If you're not familiar with the EDP data types, we have attributes of members, activities, and events. Any of those can be selected as the target outcome that we are predicting with this propensity model. So, let's provide an example here of predicting a future activity. In this case, I want to look at purchase. So, system activity for point of sale transactions. A couple other things you can specify around currency, et cetera, but as well, the time period. So depending on the type of business that you have one month may make sense. If you're maybe be a grocer, maybe one week makes sense. A furniture store or a car dealership, the buying cycle is much, much longer. You can amend that to be days, weeks, or many months. Also some additional conditions if you wish to get a little bit more granular. The two for one here I'm going to show is in this same workflow, you can instead predict a member attribute and specifically to find lookalike members. You have a customer lifetime value attribute in your EDP already. You can specify or define that attribute, select here to find lookalike members, and then here again, you can get more granular with which part of the lifetime value spectrum you wish to find lookalikes for. So, let's go greater than a thousand dollars in total lifetime value. Here again, I can set the time horizon. The next step is feature selection, which is really a fancy way of saying this is all the data that I'm going to pour into the algorithm and let the machine learning sift through it and identify where there's signal and where there's static. These data points or attributes have been separated into different categories just for ease of use, but essentially you can choose to include all attributes. You can one by one, eliminate those that you don't wish to include, or you can start from scratch and only specify those that you explicitly want to include. This can be done again across attributes, events, and activities. And this is again providing the algorithm with the inputs that it can use to create groups, to understand influencing factors, predictive factors. Finally there is an advanced ML configuration step. So, this may be for the more advanced user. So again, with feature selection, we're specifying the inputs that the model can use to determine where there is predictive power or an influencing factor by a given attribute, event or activity. Finally, we have an advance step for machine learning configurations. For the more sophisticated user, or one which might have a data scientist colleague down the hall that they can tap the shoulder of, here are some advanced settings or parameters that can be specified per the data scientist's desires. One example here is to amend our default setting for removal of missing values or fields with missing values, I should say. So, data scientists may know that there are some inputs that are really highly predictive of given outcomes based on models they've built in the past, but not fully populated. So, may only have an 80% completion rate, whereas our default setting may have suppressed those inputs if the population rate was lower than 95%. All right, and that's it. We get to the review step. I did not talk much about member attribute mapping, but as we're iterating through model training or model building, that is not important, that only becomes important when we get to the scoring step. Going to do a quick review, make sure that I'm happy with the configuration here and save this instance. You can see my Signals21 CDS Demo Model appears here at the top of my machine learning list. Now, once this is run and I click in, I can view the insights dashboards in the very same manner that I can, any of the out- of- the box models that we provide through the Cheetah platform. So, in the case of a propensity model, I'll look at a distribution of scores, for example, 140,000 of those eligible to be modeled here, have a 60% to 65% likelihood to purchase in this case. And then down here, as I've referenced numerous times, you can explore the predictive factors. So again, understanding what the influencers are of a given outcome is as important as having a score appended to a member record. I can also look at migration patterns. Once the model has been scored multiple times, I can look to see whether the high propensity to purchase group is migrating toward moderate or low propensity and vice versa, and I can look at a wider historical trend as well. So not just from one scoring run to the next, but over a four, five, six month period. And then finally, for the more advanced user, we are very transparent about the model performance. So those that may wish to interrogate the confusion matrix, for example, to understand the amount of predictions that were actually accurate, or true positives, or true negatives versus those that are false positives or false negatives. All right, so here we are back at the machine learning homepage. Just one last thing to point out is that while I ran through a propensity model, the Citizen can also build custom send time optimization and clustering models. In the future, we'll expand our offer recommender capability to the Citizen Data Scientist as well.
Nick Einstein: Great stuff Schuyler, who is Citizen Data Scientist for? Who do we design it for?
Schuyler Wareham: We design it for our everyday users, marketers. You the marketer, you know your business, you know what data you have available within our platform, the problems that you're trying to solve, and our CDS module can empower you to make timely decisions and learn from the insights that those models generate.
Nick Einstein: Before we go, you have some thoughts here on next steps for marketers to take when adopting AI/ ML and maybe where CDS fits in.
Schuyler Wareham: Yeah, I mean, this is more broadly in terms of adoption of AI and ML. First thing just reiterate, is learning, ingesting and digesting insights that are the outputs of these models, not just again, a score or a cluster membership designation, but the predictive factors, the profile characteristics of those groups. Next, it's really important that there are mechanisms for action that are tightly integrated with machine learning features to not keep those things siloed, grease the skids towards execution, is really important. Items three and four here are really where the Citizen Data Scientist comes in. The third step, you can really in a more simple way, just augment existing models. In the fourth, you can build custom models that are more fully bespoke to your use cases and your data. And then fifth, we have an upcoming feature, will be launched soon, and we call it Expert Portal where an expert data scientist can integrate their Jupyter and Python tools and embed any custom, fully bespoke models in our platform, operationalize those models and again, grease the skids towards action.
Nick Einstein: Thanks for joining us today. If you'd like to learn more about Citizen Data Scientist, check out the CDS help center, or reach out to your customer success rep.
DESCRIPTION
Most marketers do not have enough data science resources at the ready to efficiently extract predictive and prescriptive insights from their customer data. Join this session led by Nick Einstein and Schuyler Wareham from the product team to learn how to democratize your data and enable more of your marketing team members to generate insights and audiences without having deep knowledge of data science and advanced analytics.
Today's Guests
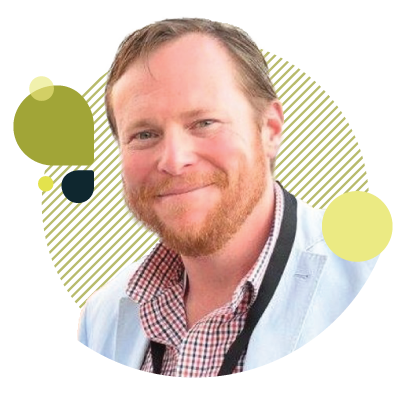
Nicholas Einstein
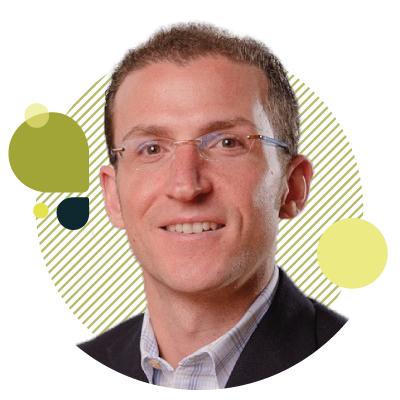